Pecan’s CEO and co-founder Zohar Bronfman delivered a keynote talk at the 2023 D3 Conference, the technology and innovation conference organized by UST. This is a lightly edited transcript of his talk.
I titled my presentation “Prediction is All You Need.” It’s a reference to “Attention Is All You Need,” the seminal paper that is basically the foundation for large language models, the GenAI models we are talking about. I’m alluding to the fact that there’s an element missing with GenAI today. And I’ll explain more as we go.
A little bit about myself: I’ve been doing AI for 15 years now in academia and industry. In academia, mostly at Tel Aviv University, and in industry, at Pecan. A quick word about Pecan, the startup that Noam, my co-founder, and I started together.
Pecan is like a data scientist in a box. I came to the realization that artificial intelligence, machine learning, data science, and predictive analytics — there are many other names that we can throw out there — are amazing capabilities that businesses can and should use. We also came to realize that it’s very hard to implement those capabilities.
I want to illustrate what it means to run a good data science or AI project. Let’s look at this example. Let’s call this person John. John is having a conversation with the support center of his cable provider. You don’t need to be a data scientist to predict that John is going to churn, but you might have been able to predict that churn a month in advance. You could have known that this was going to escalate.
As the cable provider, you could have preempted this behavior by, for example, providing John with a promotion a month ahead, making him more comfortable with the cable provider he’s subscribing to. That’s the world of data science, transactional data science, or predictive analytics. There are many names out there.
Let’s demystify what happens under the hood. Traditionally, you have data within organizations: proprietary data, transactions, events, promotions, interactions with the call center, and many other types of data. Those have to be collated, aggregated, and prepared for machine-learning algorithms. Then, you have machine learning. It generates predictions.
For example, John is going to churn next month. And then, based on those predictions, you act to retain John from churning, for instance. Pecan is wrapping all of these capabilities into an automated box. That’s what we are doing.
GenAI came roughly a year ago into the center of the stage and made AI something everyone’s talking about. And make no mistake, we all believe GenAI is amazing. It’s so amazing that it can actually pass an MBA exam at Wharton. That might also say something about MBA exams at Wharton — but that’s a different topic.
ChatGPT can actually guide your adventure in D&D — in Dungeons and Dragons. And here’s probably the most amazing image I’ve ever seen. You can go and meet Picasso and ask him to create your Picasso-style portrait. How mind-blowing.
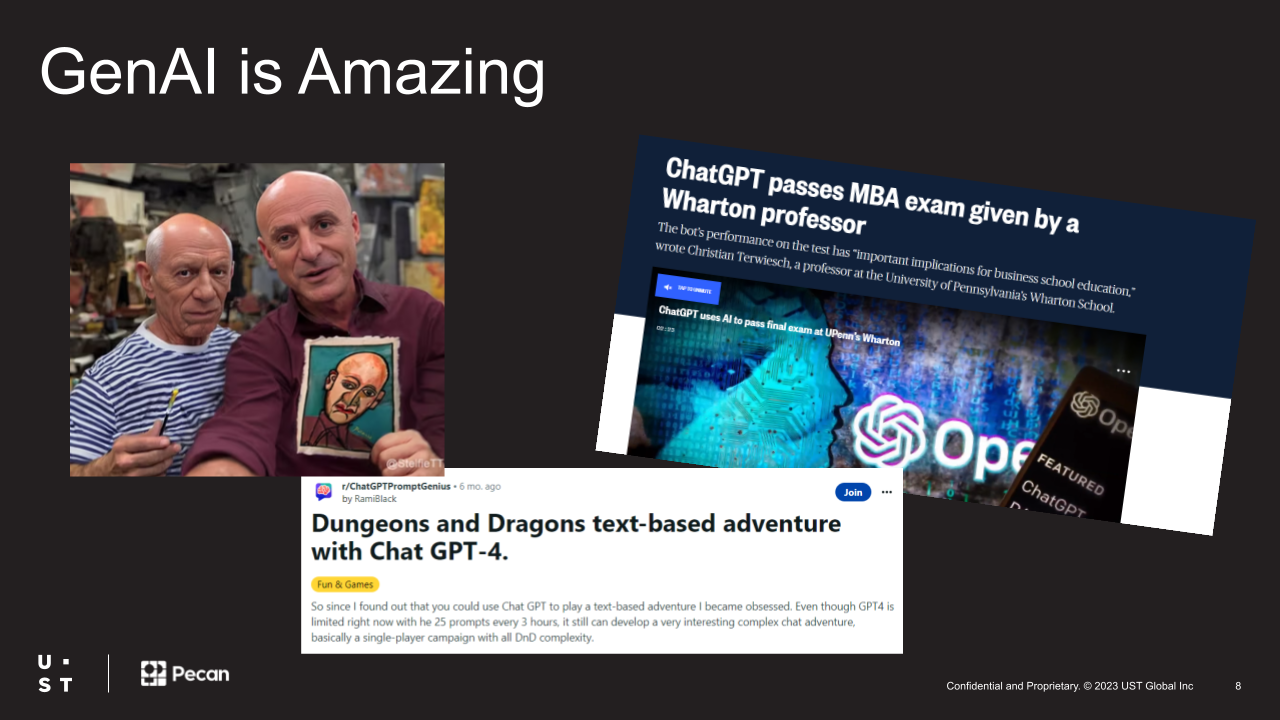
So, yes, GenAI is amazing. But what happened in the last few years that prepared everything for the emergence of such a fantastic technology? So, first of all, these state-of-the-art modeling capabilities developed over time. There is an immense amount of data out there.
But mind you, the publicly available data is mostly text and images or videos. There aren’t many business or transactional datasets [available for training models]. And that’s a crucial point for our discussion today.
Lastly, the compute — the availability and the commoditization of compute. It’s just so accessible that everyone can play with these types of models, and that’s amazing as well.
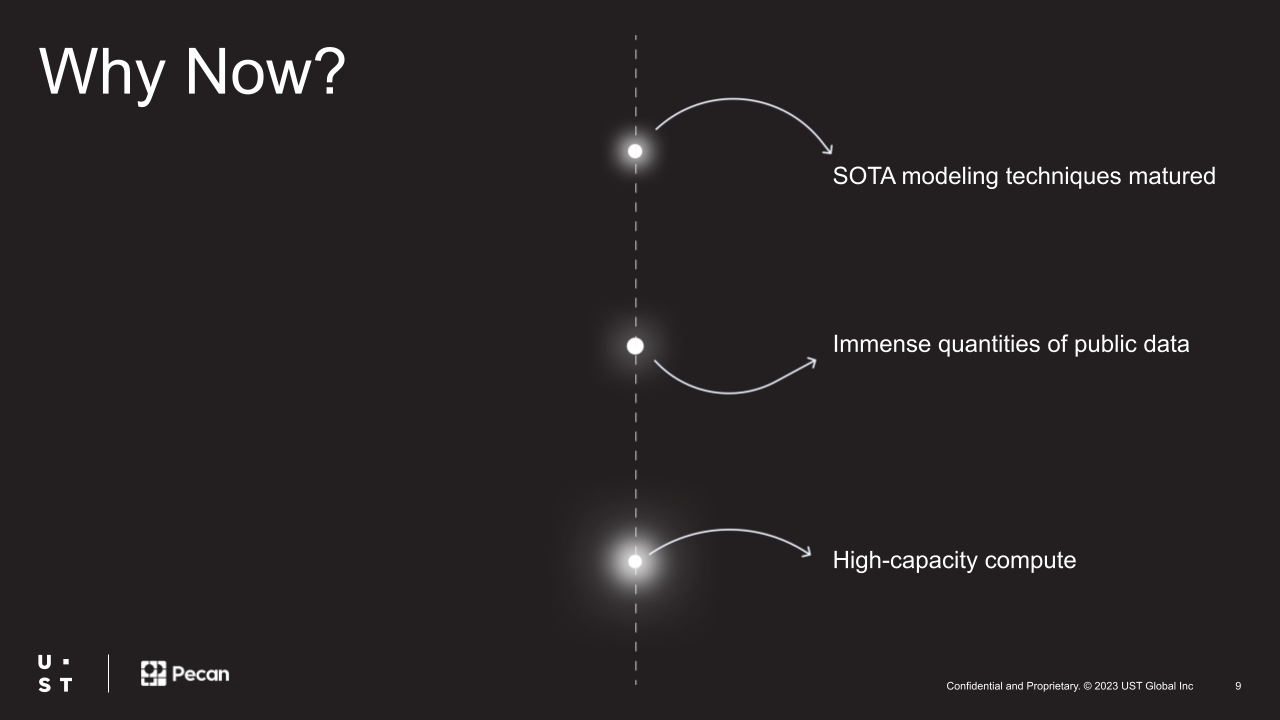
There’s one more reason why I think GenAI is so mind-blowing. I call it the “grandmother test.”
In the past, if you would try to explain to my grandmother what I was doing for a living, she was puzzled. She couldn’t get it. But if you put a grandmother in front of ChatGPT, she gets it. She gets it immediately, and it’s mind-blowing for her, too. There’s a fantastic effect for AI from this type of grandmother effect, for sure.
And relatedly, there’s a phenomenon called the “uncanny valley.” “Uncanny valley” means that we, as humans, have an aversion to machines that try to emulate or mimic human behavior that is close to human behavior but is not exactly human behavior.
And that was the case for chatbots — up until ChatGPT. We feel comfortable with machines that are very dissimilar to humans, and we feel comfortable with machines that are very similar to humans. And for the first time, ChatGPT was a bot that felt like a human. So, we are emotionally more receptive.
All of that created a huge hype and buzz and obsession. You can see the billions of dollars invested only by VCs, venture capitalists, in the last years. You can see that in 2023, there are three or four times more investments than in 2022, and we still have a quarter. The numbers go through the roof, which is amazing.
But I constantly think about this quote from Bjarne Stroustrup. He’s the inventor of C++. He said, “The only thing that grows faster than computer performance is human expectation.”
The buzz is incredible. The investment is huge. The expectations are out there. Now it’s delivery time.
And there’s a question. There’s a question of whether GenAI can meet the expected delivery. We’re in a situation now where we realize that we have a huge hammer, but I’m not sure that we have a good understanding of the huge nails that go with it.
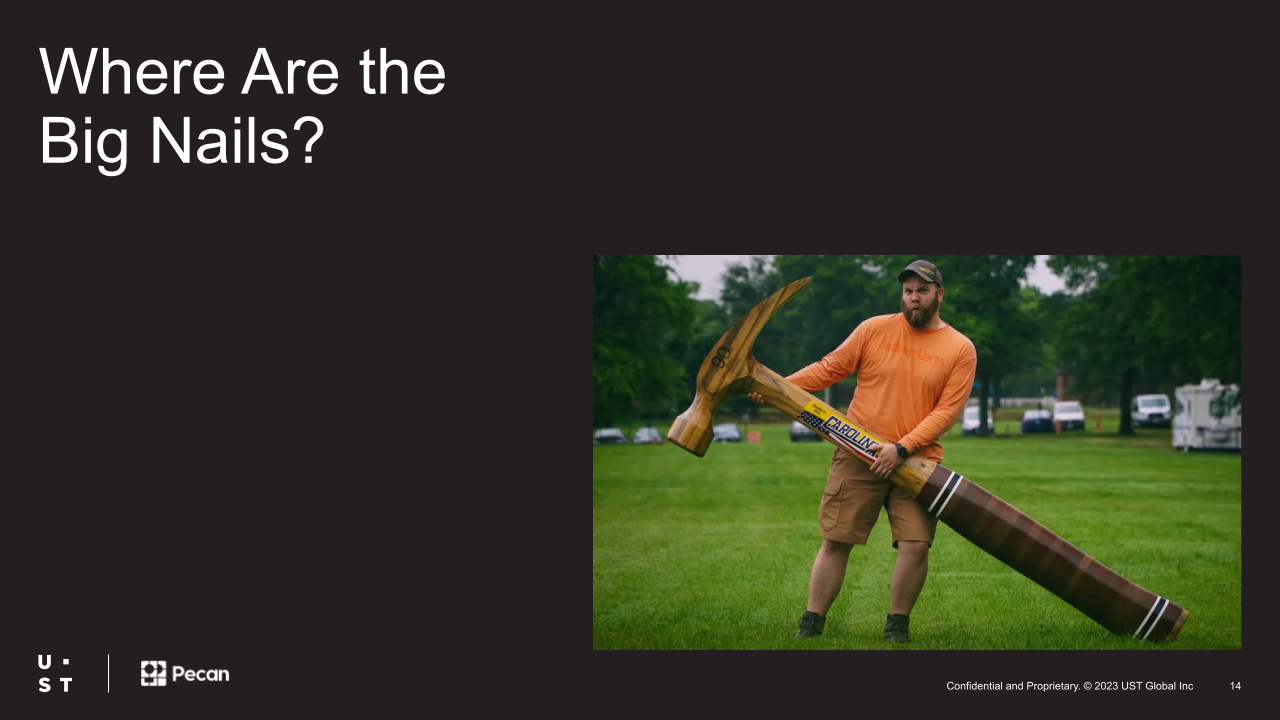
There are challenges for actually driving business value with GenAI. I’m going to name a couple of challenges that are often discussed today. And then I’m also going to introduce one more challenge that is a little bit less discussed.
The first challenge is the accuracy of these models and the hallucinations they create. In all honesty, the real problem is not the hallucinations per se. It’s the lack of so-called “resolution.” Resolution means that the model knows when it knows — and when it doesn’t know. In other words, the model can be hallucinating but also be very confident about it. It’s not a unique property of models alone. Some people also have this unique property. But with models, it’s a big issue.
When a model tells you with great certainty something completely wrong, you find yourself in a challenging place. I’ll give you just one example of a significant limitation large language models have today: They can easily learn that A is B, but they cannot learn the reverse, that B is A.
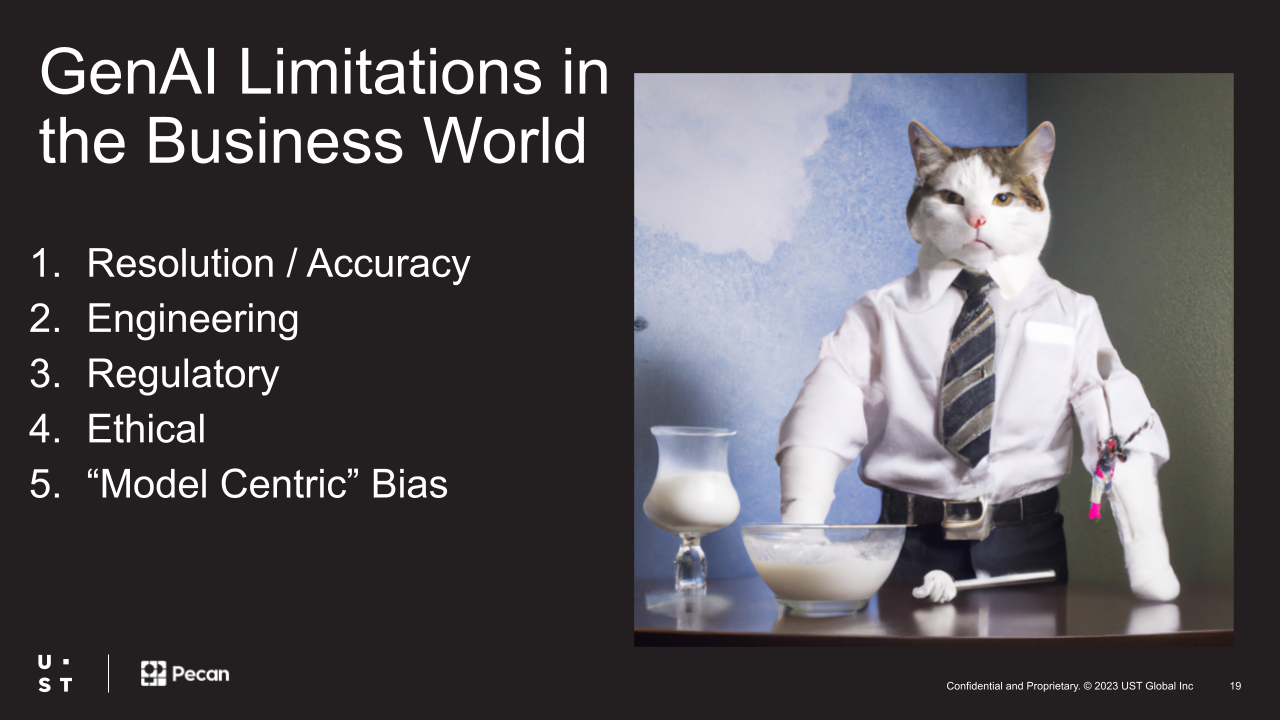
For example, it will be easy for them to learn that Camilla is Charles’s wife, but if you ask them who Camilla’s husband is, they won’t be able to make the reverse inference. However, they can tell you some weird story and be very confident about it.
The other challenge is with pure engineering. We spoke about compute earlier and GPUs. There’s a famous tweet about the fact that the Googles and the Microsofts of the world are buying all the GPUs they can, leaving few for startups or other companies. It will be hard to create LLMs on your own, but it’s also very hard to deploy LLMs. It’s also hard to make them work at scale. There are real engineering challenges that are being dealt with.
There are also regulatory questions and a lot of uncertainty around what is going to be the regulation. I have to explain this image, which was obviously created with GenAI. This is a famous Israeli idiom: “You cannot expect the cat to guard the cream.” And today, unfortunately, when it comes to regulation, most of the regulation is being done by the companies themselves. Until governments and other parts of society take hold of regulation, the cream is not entirely safe.
And last but not least of those classic limitations, there’s the ethical question. Some people think that Skynet might be around the corner. I’m not sure GenAI is as risky as people might think, but definitely, there are questions about deepfakes, questions about manipulation, and questions about data and privacy.
I want to introduce another challenge for bringing real business value with generative models. I call this challenge a model-centric bias. What I mean by model-centric bias is that you focus on one easy, relatively easy measure that is sexy and fun to optimize for, but it doesn’t really solve the whole situation.
I’ll give you an example from the world of cars. If you are a car manufacturer, it’s very, very fun and exciting to build the fastest car on the planet. I’m excited by seeing very, very fast cars. But if you ask about how I can generate revenue from cars, it’s clear that you need something more holistic. You need a car that is safe. You need a car that is cost-efficient. You need a car that is comfortable. Obviously, the SUV is not that sexy, but it solves a more holistic problem and, therefore, generates all of the revenue.
I’m arguing that with GenAI today, we’re still at the phase where we are very model-centric and looking into the cool aspects of what the models can do — instead of asking ourselves how to incorporate GenAI into a more holistic, problem-centric approach.
The good news is that it’s not the first time this has happened. Data science was model-centric for many, many years before. And it’s one of the major reasons close to 90 percent of data science projects failed. It’s a crazy number, and much of it was caused by putting the focus on the model instead of the focus on the problem.
We, as a community and an industry, are maturing towards a more problem-centric and data-centric approach. Here, you can see that the estimation that the value that would be created from regular AI is going to be huge, compared to pure GenAI isolated from the business environment.
I want to offer a potential “big nail” for GenAI that can be exciting for many of us. I think of ML and GenAI as weird twins. They can work quite well together. In some sense, machine learning, or more classical AI, and GenAI need each other to create meaningful business value.
In my opinion, generative models — large language models — are very poor at working with tabular data. That’s the take-home message.
The available data out there is textual and image. They are trained on that kind of data. And when it comes to proprietary business data, they perform poorly.
ML is the exact opposite. ML performs amazingly well on tabular data. If we can combine them, we can benefit from the advantages of each of those AI twins.
I want to leave you with one thought. This is a plausible future conversation a CEO could have with a bot or agent.
The CEO will ask, “What’s the expected churn rate in Massachusetts next month?” The agent will answer: “It will be between 7.3 and 7.9 percent.”
The CEO, surprisingly, will ask, “Can we reduce it to seven?”
And the bot will say, “Yes, with 85 percent likelihood and a cost of $1 million. It will require personalized gifting, but you’re expected to save at least $2 million from the extra retention dollars, representing 100 percent IRR — internal rate of return — within our threshold of operations. May I execute?”
By combining the GenAI capabilities of personalization, of creative, of ingenuity when it comes to customer interactions — along with the tabular capabilities of AI and machine learning that can provide these predictions around business performance — we will be able to achieve such a conversation. I believe this is what will move the needle for businesses.
Thank you very much.