Challenge
This mobile game maker publishes over a dozen games, and their cutting-edge concepts and gameplay draw millions of players daily. One of their hit series — real-time strategy games — has over 100 million downloads, but a few years after its release, the flagship game of the series started to lose momentum.
The team had previously used a sophisticated lower-funnel user acquisition strategy based on App Event Optimization (AEO) and Value Optimization (VO) techniques. However, this user acquisition approach is optimized on post-click conversion data up to day 7.
In just 7 days, many users haven’t accomplished much in a game, nor have they spent much money. That means there are relatively few users and data to pass to Meta for optimization and user acquisition for new campaigns. The lack of data limited the ability to grow the user base for this game.
To advance their lower-funnel strategy, the UA team wanted a way to identify more high-value, enthusiastic users who would be likely to convert beyond Day 7 and whose acquisition would be more cost-effective.
Solution
Pecan’s platform connected to the game publisher’s BigQuery database to retrieve data for automated prep and modeling, initially focusing on Android user data. Pecan constructed models to predict players’ activity and spend at Day 30 of their activity, based on their interaction with the game from installation until Day 2.
For each new user, the model generated a pLTV prediction and assigned a percentile score. The top 1% predictions were passed back to the game maker’s MMP, AppsFlyer. These events were then mapped from AppsFlyer to Facebook via server-to-server integration and used in Custom Event Optimization (CEO) to test the efficacy of this approach.
The process of accessing and preparing data was straightforward. In total, it took less than 2 months to complete the process and begin generating predictions that the UA team could immediately act upon.
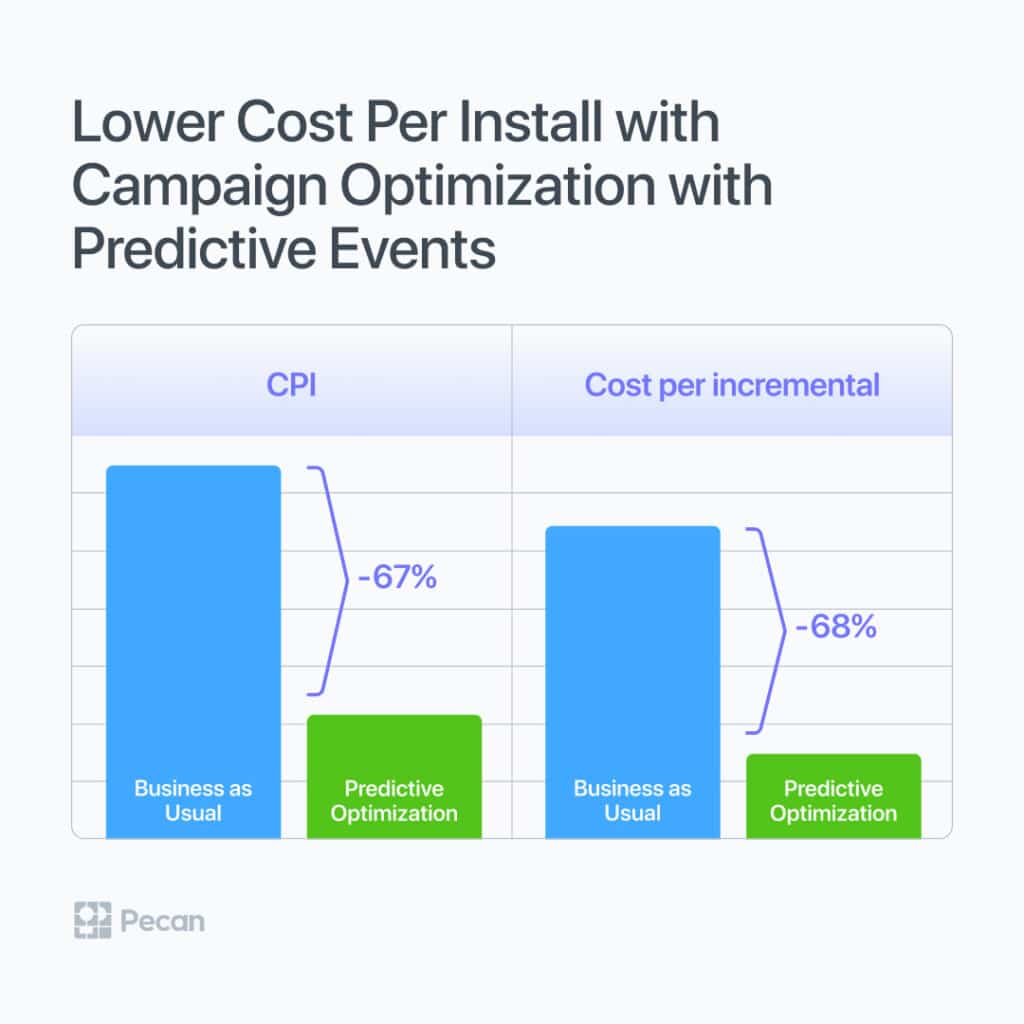
Results
Using Pecan, the game publisher implemented an AI-powered predictive analytics approach that significantly improved player spending, reduced CPIs, and led to remarkably higher ROAS.
Working with the Meta Gaming Team and Marketing Science Team, Pecan designed and executed a fair test using the Conversion Lift Study to compare the Custom Event Optimization campaign, optimized for pLTV events, and the game maker’s business-as-usual lower-funnel optimization campaigns. This test was four weeks long and included samples of U.S. users on Android devices.
The predictive CEO campaign focused on the top 1% of users, based on their pLTV. A budget was allotted to each group. The test tracked the number of app installs and the amount of purchases, as well as other metrics, including CPI and ROAS.
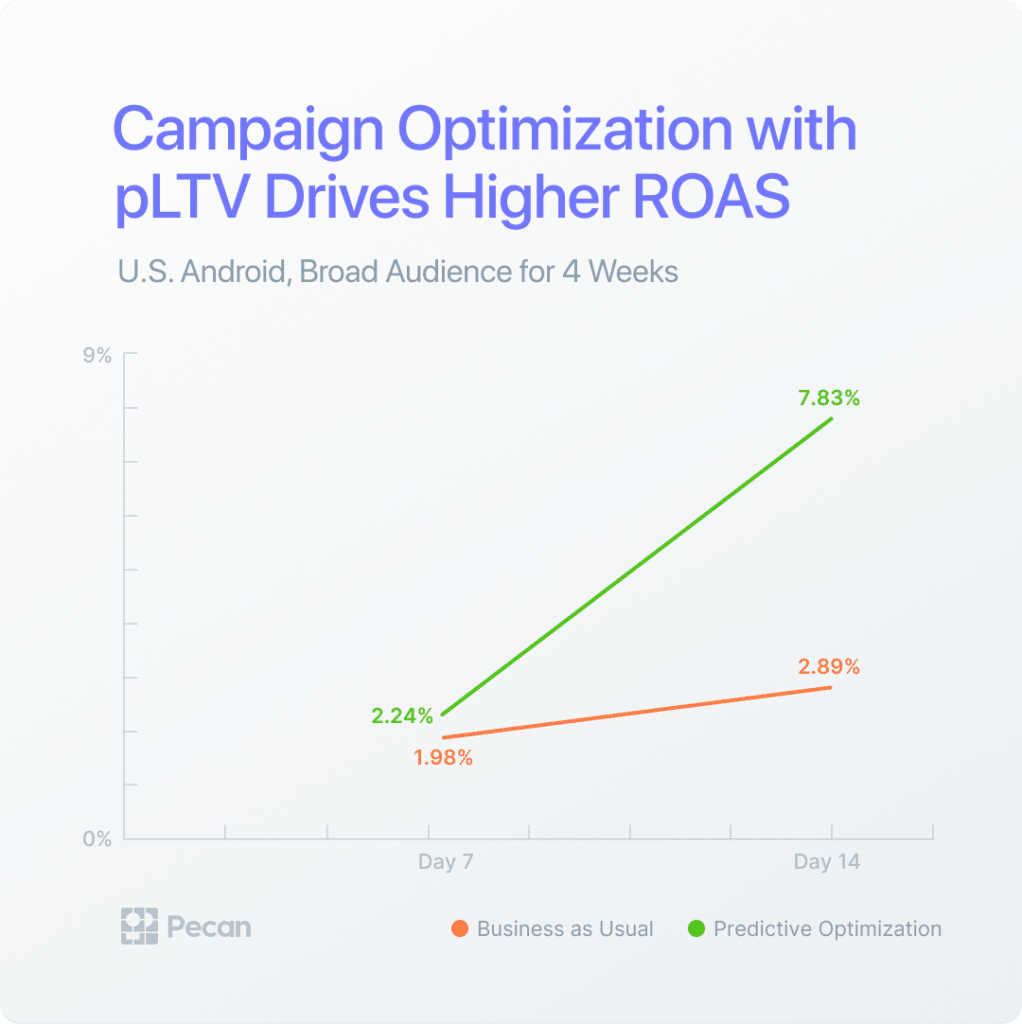
Overall, the Conversion Lift Study revealed an impressive incremental impact of using the CEO campaign optimized on pLTV events, based on Pecan’s predictions.
Compared to the BAU lower-funnel optimization, pLTV-driven CEO showed 68% lower cost per incremental install, while driving ROAS 2.7X greater than that of the players in the control group. Additionally, player spend was boosted by 34% in the Pecan group.
With Pecan’s predictions, the user acquisition team could gain higher-LTV players more often and more cheaply, with three times the number of installs in the control group — at one-third of the control group’s CPI.
Pecan’s predictions enabled more efficient identification and acquisition of high-value users—exactly the kind of positive momentum this team needed.