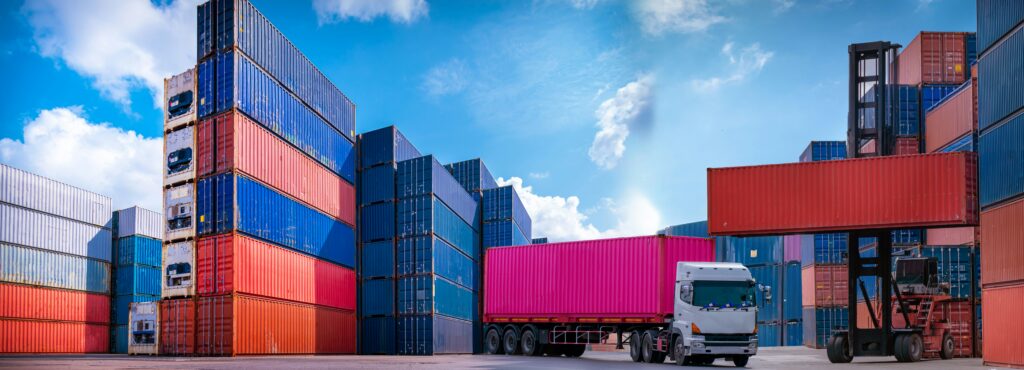
Introduction to Machine Learning and Explainability
Machine learning (ML) is transforming supply chain operations—especially in demand forecasting—by uncovering complex patterns in data to make highly accurate predictions.
For example, Walmart has successfully leveraged ML to optimize inventory levels, reduce waste, and minimize stockouts by incorporating a wide range of data inputs, from historical sales to external factors like weather.However, despite its power, ML often has a “black-box” reputation, which makes it difficult for stakeholders to understand how decisions are made and trust them.
Explainability in ML is the practice of making these model decisions transparent and interpretable. It generally falls into two categories:
- Global explainability provides insights to how the model behaves and makes decisions across all predictions.
- Local explainability focuses on why the model arrived at a specific prediction for a given input.
As outlined in this article, explainability is essential for building trust, achieving widespread adoption, and driving continuous improvement in AI-driven forecasting.
Challenges of Using an AI Demand Forecasting Model
While AI-driven demand forecasting models offer significant advantages, their adoption faces key challenges, particularly in the Integrated Business Planning (IBP) process. A fundamental tension exists between business and supply chain planning teams—business teams often present forecasts driven by growth aspirations, while planning teams focus on aligning projections with actual demand realities. Their priorities differ: business leaders aim to drive revenue and market expansion, whereas planners seek to minimize forecast error (MAPE – Mean Absolute Percentage Error), optimize inventory levels, and improve overall supply chain efficiency.
This disconnect can lead to operational inefficiencies, as business teams push for ambitious targets while planning teams emphasize data-driven realism. During IBP meetings, business teams may question why forecasts appear conservative, while planning teams must justify model projections using historical data and real-time inputs. For instance, a sales leader might advocate for higher forecasts due to an upcoming promotion, while planners highlight trends showing diminishing returns from similar campaigns in the past.
Without explainability and data-driven insights, these discussions often become contentious rather than productive, leading to misalignment in supply chain planning. This misalignment can result in stock shortages, overstock issues, and inefficiencies in decision-making, ultimately impacting the supply chain’s responsiveness and profitability. As a result, businesses frequently debate forecast credibility and ownership, highlighting the need for transparent and interpretable AI-driven forecasting.
The Importance of Explainability for Forecast Adoption
For AI forecasts to be successfully adopted, planning teams must not only trust the predictions but also be able to explain and justify them. Without clear insights into what factors drive a forecast, planning teams will struggle to align with their business counterparts. Transparency in forecasting is essential for fostering trust, facilitating collaboration, and ensuring that forecasts are both realistic and actionable.
The Challenge of ML Models in Providing Explainability
Despite their predictive power, ML models struggle with explainability due to the complexity of the patterns they identify. Techniques such as SHAP (SHapley Additive exPlanations) and LIME (Local Interpretable Model-agnostic Explanations) help address this challenge by providing insights into which features most influence a particular prediction, making AI-driven forecasts more interpretable for business users. These models often find intricate relationships in data that are difficult to articulate in a way that aligns with business intuition. This gap between ML-driven insights and human interpretability creates resistance to adoption and limits the ability of planning teams to use forecasts effectively. Traditional econometric models, in contrast, are designed with a strong emphasis on explainability, ensuring that their predictions are easily understood and justified within a business context. However, they often fall short in predictive accuracy, particularly in dynamic environments with complex, nonlinear patterns. To fully leverage AI forecasting, businesses must seek a hybrid approach that balances the transparency of traditional models with the predictive power of ML.
Local vs. Global Explainability: Why Local Matters in Demand Forecasting
While global explainability helps in understanding overarching model behavior, demand forecasting requires local explainability—a granular understanding of individual forecasts. Planning teams need to engage in meaningful discussions during IBP meetings with business teams, and for that, they must know why a specific forecast is what it is. This level of detail is necessary for aligning expectations and ensuring the forecast is actionable at the operational level.
Understanding Features in ML Models
For non-data scientists, features in ML models are the input variables used to make predictions. These can include historical sales data, promotional activities, seasonal trends, and external factors like weather or economic conditions. The quality and relevance of these features determine the accuracy of forecasts. To make AI forecasting useful in business settings, features must be designed to capture real-world patterns in a meaningful way.
Bridging the Gap Between Features and Business Understanding
A critical challenge in AI demand forecasting is translating ML features into business-relevant explanations. For example, an ML model may identify “sales velocity shifts due to regional promotions” as a key feature. While this is a technical insight, it must be translated into a business-relevant driver such as “recent marketing campaigns in the Midwest are driving increased demand for Product X.” This ensures that planning teams and business leaders can interpret the forecast in a meaningful way and take informed actions. Business users need forecasts explained in terms they understand—such as promotions, market conditions, or competitor actions—rather than technical data points. To achieve this, features must be categorized into business drivers that clearly communicate the rationale behind a forecast in a way that aligns with business intuition. Only by framing AI-driven insights in these business-relevant terms can companies foster trust, enhance adoption, and drive tangible value from demand forecasting models.
Conclusion
A truly effective AI demand forecasting solution demands more than just accuracy—it must also provide local explainability in business terms. By investing in explainable AI tools, training planning teams to interpret ML outputs, and baking transparency into IBP discussions, organizations can bridge the gap between aspirational sales targets and operational realities.
This alignment leads to more accurate forecasts, smoother collaboration, and ultimately a more resilient, profitable supply chain.
Ready to elevate your demand forecasting?
Our platform not only delivers accurate forecasts but also provides clear, localized explanations you can trust. Don’t let demand uncertainty slow you down.
Get in touch and discover how Pecan can help your organization forecast smarter and act faster.